Keynotes

Na Li (Harvard)
Representation-based Learning and Control for Dynamical Systems
Tuesday, July 16, 09:15 - 10:00
Abstract: The explosive growth of machine learning and data-driven methodologies have revolutionized numerous fields. Yet, the translation of these successes to the domain of dynamical physical systems remains a significant challenge. Closing the loop from data to actions in these systems faces many difficulties, stemming from the need for sample efficiency and computational feasibility, along with many other requirement such as verifiability, robustness, and safety. In this talk, we bridge this gap by introducing innovative representations to develop nonlinear stochastic control and reinforcement learning methods. Key in the representation is to represent the stochastic, nonlinear dynamics linearly onto a nonlinear feature space. We present a comprehensive framework to develop control and learning strategies which achieve efficiency, safety, robustness, and scalbility with provable performance. We also show how the representation could be used to close the sim-to-real gap. Lastly, we will briefly present some concrete real-world applications, discussing how domain knowledge is applied in practice to further close the loop from data to actions.
Bio: Na Li is a Winokur Family Professor of Electrical Engineering and Applied Mathematics at Harvard University. She received her Bachelor's degree in Mathematics from Zhejiang University in 2007 and Ph.D. degree in Control and Dynamical systems from California Institute of Technology in 2013. She was a postdoctoral associate at the Massachusetts Institute of Technology 2013-2014. She has held a variety of short-term visiting appointments including the Simons Institute for the Theory of Computing, MIT, and Google Brain. Her research lies in the control, learning, and optimization of networked systems, including theory development, algorithm design, and applications to real-world cyber-physical societal system. She has been an associate editor for IEEE Transactions on Automatic Control, Systems & Control Letters, IEEE Control Systems Letters, and served on the organizing committee for a few conferences. She received the NSF career award (2016), AFSOR Young Investigator Award (2017), ONR Young Investigator Award(2019), Donald P. Eckman Award (2019), McDonald Mentoring Award (2020), the IFAC Manfred Thoma Medal (2023), along with some other awards.
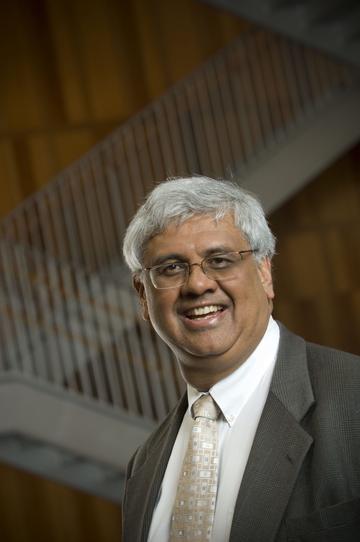
S. Shankar Sastry (UC Berkeley)
Learning Enabled Multi-Agent Systems in Societal Systems Transformation
Tuesday, July 16, 11:30 - 12:15
Abstract: Opportunities abound for the transformation of societal systems using new technologies and business models to address some of the most pressing problems in diverse sectors such as energy, transportation, health care, manufacturing and financial systems. Most notably, the integration of CPS, and AI/ML. The issues of transforming societal systems is accompanied by issues of economic models for transformation, privacy, (cyber) security and fairness considerations. Indeed, the area of “mechanism design” for societal scale systems is a key feature in transitioning the newest technologies and providing new services. Crucially, human beings interact with automation and change their behavior in response to incentives offered to them. Training, Learning and Adaptation in Human AI Teams (HAT) is one of the most engaging problem in Human-AI/ML systems today. In this talk, I will present a few vignettes: how to align societal objectives with Nash equilibria using suitable incentive design, proofs of stability of decentralized decision making while learning preferences. The application of the techniques to problems in road transportation, Advanced Air Traffic Management and other societal scale systems will also be presented. The work is joint with Chinmay Maheshwari, Manxi Wu, Kshitij Kulkarni, and Pan Yang Su.
Bio: S. Shankar Sastry was the Dean of Engineering from 2007-18. He is currently the co-Director of the C3 Digital Transformation Institute (C3DTI), an institute aimed to develop the science and technology of digital transformation in societal systems. He is also the director of the FHL Vive Center for Enhanced Reality for exploring the boundaries between augmented reality and real scenes with applications to autonomy, performance arts, and education. He is deeply committed to the use of technology to lift people out of poverty through his directorship of the Blum Center for Developing Economies 2007-22.
He has coauthored about 700 technical papers. He has co-authored or co-edited 10 books, including Adaptive Control: Stability, Convergence and Robustness (with M. Bodson, Prentice Hall, 1989) and A Mathematical Introduction to Robotic Manipulation (with R. Murray and Z. Li, CRC Press, 1994), Nonlinear Systems: Analysis, Stability and Control (Springer-Verlag, 1999), and An Invitation to 3D Vision: From Images to Models (Springer Verlag, 2003) (with Y. Ma. S. Soatto, and J. Kosecka)., and Generalized Principal Component Analysis (Springer Verlag, 2016 with R. Vidal and Y. Ma). Dr. Sastry was elected to the National Academy of Engineering in 2001 the American Academy of Arts and Sciences in 2004. He received the President of India Gold Medal in 1977, the NSF Presidential Young Investigator Award in 1985. He got the Eckman Award of the American Automatic Control Council in 1990, the Ragazzini Award for Distinguished Accomplishments in teaching in 2005 and the Rufus Oldenburger Career Award of ASME in 2021. He has received honorary doctorates from KTH, the Royal Institute of Technology, Stockholm, a Ph.D. Honoris causa from the University of Waterloo, Canada, and a Laurea Dottorato honoris causa from Politecnico di Torino, and the Berkeley Citation.
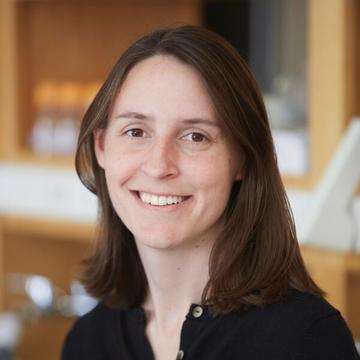
Mary Dunlop (Boston University)
Optogenetic Feedback Control of Gene Expression in Single Cells
Tuesday, July 16, 14:30 - 15:15
Abstract: In this talk I will discuss a novel approach for controlling gene expression dynamics in single cells that can be used to precisely drive expression in thousands of cells in parallel. Using recent advances in the fields of machine learning and control theory, we train a deep neural network to accurately predict the response of an optogenetic system in E. coli cells. We then use the network in a deep model predictive control framework to impose arbitrary and cell-specific gene expression dynamics on thousands of single cells in real time, applying the framework to generate complex time-varying patterns. We also showcase the framework’s ability to link expression patterns to dynamic functional outcomes by controlling expression of an antibiotic resistance gene. These approaches offer powerful methods that can be used to quantify and control cell-to-cell heterogeneity in antibiotic resistance, providing a detailed view into strategies bacteria can use to evade drug treatment.
Bio: Mary Dunlop is an Associate Professor of Biomedical Engineering at Boston University with additional appointments in Bioinformatics and in the Molecular Biology, Cell Biology & Biochemistry program. She graduated from Princeton University with a B.S.E. in Mechanical and Aerospace Engineering and a minor in Computer Science. She then received her Ph.D. from the California Institute of Technology, where she studied synthetic biology with a focus on dynamics and feedback in gene regulation. Her lab engineers novel synthetic feedback control systems and studies naturally occurring examples of feedback in gene regulation. In addition, her research has focused on understanding the role of cell-to-cell heterogeneity in bacterial systems. In recognition of her outstanding research contributions, she has received many honors including election as an AIMBE Fellow, the NSF Transitions Award, ACS Synthetic Biology Young Investigator Award, DOE Early Career Award, and NSF CAREER Award. She is also the recipient of several teaching awards, including Boston University’s Biomedical Engineering Professor of the Year Award and the College of Engineering Teaching Excellence Award.
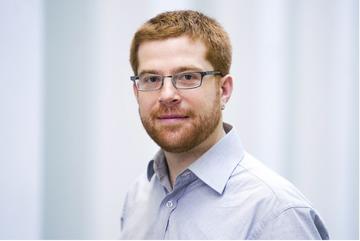
Jonas Buchli (DeepMind)
The state of optimal and learning control in the 2020s
Wednesday, July 17, 09:00 - 09:45
Abstract: The last decade has brought tremendous progress in our ability to solve very complex sequential decision making problems. This progress is enabled by building on the principle of optimality, leveraging advances in automatic differentiation, nonlinear optimization and increased hardware and software compute and storage performance. The leap in capability has given us a different perspective on some central notions of control engineering, such as ‘control vs planning’, ‘control objective’, ‘models’ among others and how we operationalize these concepts. In particular, it allows, but also necessitates us to focus more and more on the ‘What’ rather than the ‘How’ to control, i.e. a focus on formulating control challenges and objectives rather than their actual solution as well as their embedding within the larger technical systems. In this talk I will review some of the central concepts and developments behind this progress and show some intriguing examples of this conceptual shift at work.
Bio: Jonas Buchli is a Senior Research Scientist with Google Deepmind, London. He holds a Diploma in Electrical Engineering from ETH Zurich (2003) and a PhD from EPF Lausanne (2007), Switzerland. He has been working at the intersection of Machine Learning and Control for most of his career. He has been a contributor to a variety of interdisciplinary research projects in Disaster Assistance, Architecture, Biomedical Technology, and Paleo-anthropology among others.

Jan Peters (Technical University of Darmstadt)
Inductive Biases for Robot Reinforcement Learning
Wednesday, July 17, 11:15 - 12:00
Abstract: Autonomous robots that can assist humans in situations of daily life have been a long standing vision of robotics, artificial intelligence, and cognitive sciences. A first step towards this goal is to create robots that can learn tasks triggered by environmental context or higher level instruction. However, learning techniques have yet to live up to this promise as only few methods manage to scale to high-dimensional manipulator or humanoid robots. In this talk, we investigate a general framework suitable for learning motor skills in robotics which is based on the principles behind many analytical robotics approaches. To accomplish robot reinforcement learning learning from just few trials, the learning system can no longer explore all learn-able solutions but has to prioritize one solution over others – independent of the observed data. Such prioritization requires explicit or implicit assumptions, often called ‘induction biases’ in machine learning. Extrapolation to new robot learning tasks requires induction biases deeply rooted in general principles and domain knowledge from robotics, physics and control. Empirical evaluations on a several robot systems illustrate the effectiveness and applicability to learning control on an anthropomorphic robot arm. These robot motor skills range from toy examples (e.g., paddling a ball, ball-in-a-cup) to playing robot table tennis, juggling and manipulation of various objects.
Bio: Jan Peters is a full professor (W3) for Intelligent Autonomous Systems at the Computer Science Department of the Technische Universitaet Darmstadt since 2011, and, at the same time, he is the dept head of the research department on Systems AI for Robot Learning (SAIROL) at the German Research Center for Artificial Intelligence (Deutsches Forschungszentrum für Künstliche Intelligenz, DFKI) since 2022. He is also is a founding research faculty member of the Hessian Center for Artificial Intelligence. Jan Peters has received the Dick Volz Best 2007 US PhD Thesis Runner-Up Award, the Robotics: Science & Systems - Early Career Spotlight, the
INNS Young Investigator Award, and the IEEE Robotics & Automation Society's Early Career Award as well as numerous best paper awards. In 2015, he received an ERC Starting Grant and in 2019, he was appointed IEEE Fellow, in 2020 ELLIS fellow and in 2021 AAIA fellow.
Despite being a faculty member at TU Darmstadt only since 2011, Jan Peters has already nurtured a series of outstanding young researchers into successful careers. These include new faculty members at leading universities in the USA, Japan, Germany, Finland and Holland, postdoctoral scholars at top computer science departments (including MIT, CMU, and Berkeley) and young leaders at top AI companies (including Amazon, Boston Dynamics, Google and Facebook/Meta).
Jan Peters has studied Computer Science, Electrical, Mechanical and Control Engineering at TU Munich and FernUni Hagen in Germany, at the National University of Singapore (NUS) and the University of Southern California (USC). He has received four Master's degrees in these disciplines as well as a Computer Science PhD from USC. Jan Peters has performed research in Germany at DLR, TU Munich and the Max Planck Institute for Biological Cybernetics (in addition to the institutions above), in Japan at the Advanced Telecommunication Research Center (ATR), at USC and at both NUS and Siemens Advanced Engineering in Singapore. He has led research groups on Machine Learning for Robotics at the Max Planck Institutes for Biological Cybernetics (2007-2010) and Intelligent Systems (2010-2021).

Shimon Whiteson (Oxford)
Efficient & Realistic Simulation for Autonomous Driving
Wednesday, July 17, 14:00 - 14:45
Abstract: In this talk, I will discuss some of the key challenges in performing efficient and realistic simulation for autonomous driving, with a particular focus on how to train simulated agents that model the human road users, such as cars, cyclists, and pedestrians who share the road with autonomous vehicles. I will discuss the need for distributionally realistic agents and describe methods for training hierarchical agents to this end. I will also discuss how to model the safety-critical events that are crucial to simulate despite rarely appearing in the data. Finally, I will discuss how the resulting simulator can be used to efficiently train a planning agent to control the autonomous vehicle itself.
Bio: Shimon Whiteson is a Professor of Computer Science at the University of Oxford and a Senior Staff Research Scientist at Waymo. His research focuses on deep reinforcement learning and learning from demonstration, with applications in robotics and video games. He completed his doctorate at the University of Texas at Austin in 2007. He spent eight years on the faculty at the University of Amsterdam before joining Oxford in 2015. His spinout company Latent Logic was acquired by Waymo in 2019.